DATA ANALYTICS
The high computational capacity available to society today, combined with good algorithms, can be applied to mineral exploration and can accelerate the complete multidisciplinary-database evaluation. The data-driven machine learning can determine dependencies between several datasets from different phases of mineral exploration, whether in 2D or 3D, defining areas of favorability in a single product. We use the random forest, neural network, clustering, XgBoost, and other algorithms on an open-source machine learning and predictive analytics platform, with distributed and in-memory processing offering massive scalability and ease of deployment of machine learning models in production.
Data-driven Machine Learning Supporting Mineral Exploration
Statistical data analysis is of fundamental importance and has been applied in mineral exploration for a long time. Nowadays, high-density information, computational capacity, and data acquisition speed allow multi-source data to be analyzed quickly. The available algorithms and training points define the interdependencies between the data, allowing characterize associations and responses from mineralizing processes and mineralized zone, preparing the next stage looking for similarities and mapping favorabilities zones. Machine learning data analysis quickly reveals features or characteristics of a mineral deposit that may take years to mature in conventional analyses. In this way, today, we see machine learning as a tool that accelerates the processes of analysis, integration, and interpretation of data from mineral exploration. We work with random forest, neural networks, and XGboost, among others, to speed up data analysis. We developed an ML platform and several data preparation functions; the application of the algorithms and the results are extraordinary; we can save several responses from mineral deposits in a library that can be applied anywhere in the world. We are available to offer pilot projects to publicize our platform and show the potential of applying machine learning in multi-scale mineral exploration.
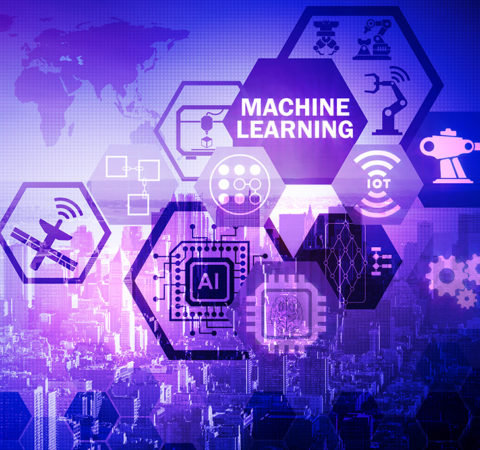